Physical Law Learning
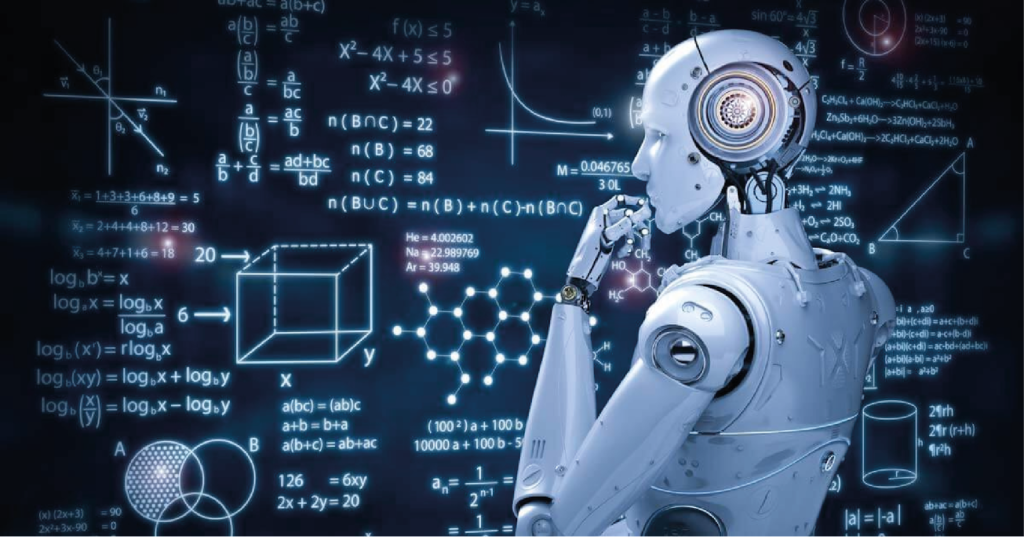
Physical models for motion and environment ensure provable trustworthy robot actions. However, already for a very specific task, the complexity of nature requires a strong learning component; even more so when taking the fact into account that next generation robots will constantly have to adapt to new tasks and scenarios, requiring lifelong learning. Thus, a key question which goes even far beyond robotics is how to optimally combine physical and learnt models. This includes intriguing challenges such as asking to which extent we can actually learn physical models. The ultimate goal will be a seamless integration and optimal combination of physical and learnt models in next generation robotics, while ensuring provable trustworthiness in terms of, in particular, robustness, safety, and explainability.
Mathematical Frameworks for Trustworthiness in Learning-facilitated Estimation & Control
Supervising PIs
Contributing PIs
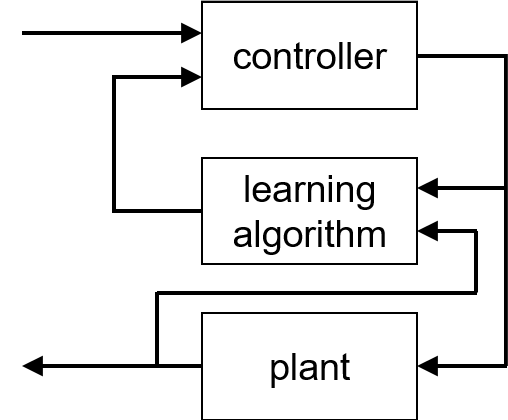
Autonomous systems need to perform goal-oriented tasks in uncertain and open-ended environments. Machine learning techniques have demonstrated tremendous successes in the context of perception and control. Still, there is a significant gap between the desired robustness guarantees in particular in the context of safety-critical systems and the often adhoc applied strategies. In this project we aim to develop novel mathematical frameworks for trustworthy learning-facilitated estimation and control of autonomous systems in uncertain environments. We will investigate fundamental limitations of learning-based estimation and control including breakdown under uncertainty, the role of architectures, and algorithmic decidability. We will further develop constructive techniques for trustworthy AI-enabled control.